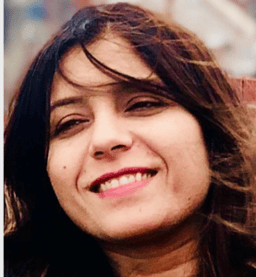
AMA: Gainsight Senior Director of Customer Success, Meenal Shukla on AI in Customer Success
July 2 @ 10:00AM PST
View AMA Answers
Gainsight Senior Director of Customer Success • July 3
To approach the initial strategy and roadmap for integrating AI into our customer success function at Gainsight, we followed these steps: 1. Assessment and Goal Setting: Identify Needs and Pain Points: Conducted a thorough assessment of our current customer success processes to identify areas where AI could add value. Key pain points included meeting prep, recording notes on customer interactions, data analysis, customer segmentation, and proactive customer strategy. Set Clear Objectives: Established clear goals for the AI integration, such as improving customer retention, increasing efficiency, and enhancing predictive analytics. 2. Stakeholder Engagement: Involve Key Stakeholders: Engaged with key stakeholders, including customer success managers, leaders, product, third-party experts, services, sales, strategy, and support to gather input and ensure alignment with organizational objectives. Customer Feedback: Collected customer feedback to understand their expectations and how AI could better serve their needs. 3. Technology Selection: Research and Evaluate AI Solutions: Researched various AI tools and platforms, considering factors like ease of integration, scalability, and cost. This involved evaluating both off-the-shelf solutions and potential custom-built options. Pilot Programs: Implemented pilot programs to test selected AI solutions on a small scale before full deployment. 4. Roadmap Development: Define Phases: Created a phased roadmap for AI integration, starting with high-impact, low-complexity projects to gain quick wins and build momentum. Resource Allocation: Allocated necessary resources, including budget, personnel, and technology, to support each phase of the roadmap. 5. Implementation and Training: Gradual Rollout: Began with the implementation of AI in data analysis and reporting, followed by more complex applications like predictive analytics and automated customer interactions. Training Programs: Developed comprehensive training programs for the customer success team to ensure they were well-versed in using AI tools effectively. 6. Monitoring and Iteration: Continuous Monitoring: Established metrics to monitor the performance and impact of AI initiatives, including AI adoption scores, stickiness scores, and retention rates. Iterative Improvement: Used feedback and performance data to make iterative improvements to the AI strategy, ensuring it remained aligned with evolving customer needs and business goals.
...Read More565 Views
1 request
Gainsight Senior Director of Customer Success • July 3
For someone who does not know what model drift is, here is the pre-read: Model drift occurs when the performance of a machine learning model degrades over time because the data it was trained on is no longer representative of the real-world data it encounters. Imagine you have a model that predicts the weather. You trained it using data from the past eight years, and it's pretty good at telling you if it will rain tomorrow based on temperature, humidity, and wind speed. This model works well initially because the weather patterns are similar to those in the training data. However, weather patterns might change over time for various reasons, such as climate change. The new weather data coming in doesn't quite match the old data the model was trained on. As a result, the model's predictions become less accurate. This mismatch between the old training data and the new real-world data is what we call model drift. Here is how we handle model drift: Continuous Monitoring: * Monitor the model's performance regularly using key metrics such as accuracy, precision, and recall. This helps identify any degradation in performance as early as possible. Data Collection and Feedback Loops: * Continuously collect new data that reflects current real-world conditions. Implement feedback loops where the outcomes of the model’s predictions are reviewed and fed back into the system Regular /Automated Retraining: * Retrain the model periodically with the latest data to ensure it adapts to new patterns and trends. This can be done on a scheduled basis (e.g., monthly or quarterly) or triggered by performance degradation or can be done on an automated basis. This can get more technical as we dive deeper. Happy to answer this question in another forum where this discussion will be more pertinent.
...Read More552 Views
2 requests
Gainsight Senior Director of Customer Success • July 3
Balancing AI-driven personalization with the need for human touch in customer interactions is crucial for maintaining strong, genuine customer relationships. Here are some of our guiding principles: 1. Define Clear Boundaries for AI and Human Interactions: * Routine Tasks for AI: Use AI to handle routine and repetitive tasks, such as data analysis, basic inquiries, and initial issue resolution. This allows customer success professionals to focus on more complex and nuanced interactions. * Human Touch for Complex Issues: Reserve human interactions for complex issues, emotional situations, and strategic discussions where empathy, understanding, and personalized attention are critical. 2. Personalized AI Interactions: * Data-Driven Personalization: Leverage AI to analyze customer data and provide personalized recommendations, insights, and content. This ensures that customers receive relevant and tailored information automatically. * Human-Like AI Responses: Design AI interactions, such as chatbots, to be conversational and empathetic, making them feel more human-like and engaging. 3. Augmenting Human Interactions with AI Insights: * Actionable Insights: Provide customer success professionals with AI-generated insights and recommendations before they engage with customers. This prepares them with relevant information and context, making their interactions more effective. * Predictive Analytics: Use AI to identify at-risk customers or opportunities for upselling, allowing customer teams to intervene proactively and with a well-informed approach. 4. Seamless Handoff Between AI and Humans: * Smooth Transitions: Ensure seamless handoffs between AI and humans. For instance, if a chatbot cannot resolve an issue, it should smoothly transition the conversation to a human without losing context. * Context Preservation: When transitioning from AI to humans, maintain the context and history of customer interactions, ensuring continuity and avoiding repetition for the customer. 5. Human Oversight and Continuous Improvement: * Monitor AI Performance: Regularly monitor the performance of AI systems to ensure they are delivering accurate and helpful responses. Use feedback to improve AI interactions continuously. * Human-in-the-Loop: Implement a human-in-the-loop approach where humans can review and override AI decisions when necessary, ensuring that AI does not make critical errors. 6. Blended Approach: * Hybrid Interactions: Combine AI and human interactions where appropriate. For example, AI can assist during a conversation by suggesting responses or actions to humans, enhancing the overall customer experience. * Co-Piloting: Use AI as a co-pilot for human agents, providing real-time assistance and suggestions while the human agent leads the interaction. 7. Transparency and Trust: * Transparent Communication: Be transparent with customers about when they are interacting with AI and when they are interacting with a human. This builds trust and sets clear expectations. * Building Trust: Ensure AI interactions are trustworthy, reliable, and aligned with the brand’s values, reinforcing customer trust in the AI systems. 8. Empathy and Emotional Intelligence: * Training for Humans: Train customer success professionals in empathy and emotional intelligence to handle interactions that require a personal touch effectively. * Empathetic AI Design: Design AI systems with a focus on empathy, using natural language processing and sentiment analysis to respond appropriately to customer emotions.
...Read More576 Views
1 request
Gainsight Senior Director of Customer Success • July 3
I am a little biased here as the company I work for (Gainsight) is a customer success solution. In the product keynote here, we explain how we are integrating AI into our tool in order to solve for six large issues in your customer lifecycle. 1. Eliminate Blindspots 2. Remove Grunt Work to Make Room for What Matters 3. Turn Every Teammate into the Best Version of Themselves 4. Make Self-Service Not Suck 5. Transform Novices into Gurus 6. Help Customers Find Their Work Bestie The first three steps in the AI Playbook are all about how your team can leverage AI to improve the entire customer journey by giving your team back time to focus on what matters. The AI playbook focuses on making it easy for customers to access the information they need to be successful and share that knowledge with others in the community. In other words, Make Self-Serve Not Suck. In the past, trainings were all about watching a video and then answering a few questions. The experience wasn’t tailored to anyone’s specific needs or use cases. AI changes all of that. With AI, customers can get a completely custom onboarding and training experience without requiring extra hours from CS teams. Customers can access trainings that are all relevant to their job and goals. The result is more engaged learning, faster understanding, and companies that quickly Transform Novices into Gurus. And the power of Human-First AI doesn’t stop at self-serve. Successful companies also use it to Help Customers Find their Work Bestie. They connect customers with similar experiences so they can build a community together. Sharing information, updating best practices, working through use cases together only makes community better and customer loyalty stronger. Everyone wins when you put humans first!
...Read More540 Views
1 request
Gainsight Senior Director of Customer Success • July 3
Still too early to answer this question but we have found that customers who adopt AI features have higher retention rate than customers who don't. AI adoption is going to be a key health score metric for us in order to drive stickiness for our product.
...Read More558 Views
1 request
Gainsight Senior Director of Customer Success • July 3
it's unlikely that companies will hire CSMs at a rate anywhere close to where they've been. As such, we need to accelerate our progress through AI toward helping companies scale without hiring additional staff. Companies are using a combination of AI and Digital CS to do 'more with more' (not more with less). So, we are seeing a trend in which companies allocate more resources to operational teams that can drive Digital CS and AI tools to make CS more efficient.
...Read More544 Views
1 request
Gainsight Senior Director of Customer Success • July 3
* Model Performance Metrics: Accuracy, Precision, Recall and some other measures here. * Operational Metrics: Latency (time taken for generating responses, the lower the latency the better), Uptime and Reliability: * Business Impact Metrics: Customer Retention Rate, NPS, CSAT * Customer Feedback and Sentiment Metrics: * Feedback Scores: Collects customer feedback on AI interactions, providing qualitative insights into the effectiveness and user satisfaction. * Sentiment Analysis: Analyzes customer sentiment from feedback and interactions, helping understand the emotional response to AI-driven services. * Economic Metrics: * Return on Investment (ROI): Evaluates the financial impact of AI initiatives, comparing the benefits derived from AI tools against their costs. * Cost Savings: Measures the reduction in operational costs due to the automation and efficiency gains provided by AI * Usage and Engagement Metrics: * Adoption Rates: Tracks the adoption of AI-driven features by customers, indicating their perceived value and usability. * Feature Utilization: Measures how frequently specific AI features are used, helping identify popular functionalities and areas for improvement
...Read More553 Views
1 request
Gainsight Senior Director of Customer Success • July 3
Here are the mechanisms we have in place: * RLHF: Reinforcement learning from human feedback (RLHF) is a machine learning (ML) technique that uses human feedback to optimize ML models to self-learn more efficiently. Reinforcement learning (RL) techniques train software to make decisions that maximize rewards, making their outcomes more accurate. * Employee Insights: Encourage customer success professionals and other employees who interact with customers to provide feedback on AI tool performance and customer reactions. * BETA Releases: Early release of AI features to select but diverse group of customers to discuss their experiences and gather qualitative feedback that can inform AI model improvements. * Sentiment Analysis Tools: Use AI-powered sentiment analysis tools to analyze customer feedback from various sources, such as emails, support tickets, and social media mentions, to gauge customer sentiment and identify common themes. * Surveys and Questionnaires: Regularly send out surveys and questionnaires to customers after interactions and at key points in their journey to gather feedback on their experiences and satisfaction. * In-App Feedback: Integrate feedback forms and rating systems within our software applications, allowing customers to provide input directly within the platform. * Customer Advisory Boards: Engage with customer advisory boards to gather in-depth feedback and insights from key customers, which can be used to refine AI models and customer success strategies.
...Read More546 Views
1 request
Gainsight Senior Director of Customer Success • July 3
* Churn Prediction: AI models can predict which customers are at risk of churn by analyzing a variety of factors, including emails, usage patterns, support ticket frequency, and customer feedback. This insight allows the team to proactively engage with at-risk customers through targeted retention strategies, personalized communication, and enhanced support, ultimately reducing churn rates. * Product Usage Trends: AI can identify trends and patterns in how customers use different product features, highlighting which features drive engagement and which are underutilized. This insight guides product development and enhancement decisions, ensuring resources are allocated to features that maximize customer value and satisfaction. * Sentiment Analysis throughout the customer journey: AI-driven sentiment analysis tools can gauge customer sentiment from various communication channels, including emails, chats, slack/team channels and support tickets. Understanding customer sentiment in real-time help in adjusting strategies promptly to address negative feedback and get cross-functional buy-in to reduce the friction points in the customer journey. * Support Ticket Resolution Time: Predictive analytics can forecast the time required to resolve different types of support tickets based on historical data. This helps in better resource planning, ensuring that the support team is adequately staffed and equipped to handle varying levels of demand, and improving response times and customer satisfaction. It also helps get buy-in from the product when you can show, through data and sentiment analysis, which problem areas are generating the most tickets. * Customer Health Scoring: AI creates dynamic customer health scores by continuously analyzing various data points, including engagement levels, product usage, support interactions, and survey responses. These health scores help prioritize which customers need immediate attention, allowing for more effective and timely interventions to improve their experience.
...Read More564 Views
1 request
Gainsight Senior Director of Customer Success • July 3
As AI becomes more integrated into the field of customer success, the role of customer success professionals is likely to evolve in several significant ways: 1. Focus on Strategic Activities: Less Routine Work: AI can handle routine tasks such as data entry, basic customer inquiries, and initial issue resolution. This frees up customer success professionals to focus on more strategic activities, such as relationship building and strategic planning. Proactive Engagement: With AI handling many operational tasks, customer success professionals can spend more time on proactive engagement, anticipating customer needs, and driving value. 2. Enhanced Decision-Making: Data-Driven Insights: AI provides advanced analytics and insights, enabling customer success professionals to make more informed decisions. This includes identifying at-risk customers, upsell opportunities, and areas for improvement. Predictive Analytics: AI-powered predictive analytics allow professionals to foresee potential issues and opportunities, making their interventions more timely and effective. 3. Improved Customer Relationships: Deeper Engagement: AI tools can analyze customer behavior and sentiment, providing insights that help customer success professionals engage more deeply with customers and build stronger relationships. Enhanced Communication: AI-powered tools like chatbots and automated email responses ensure that customers receive timely and accurate information, improving overall communication. 4. Emphasis on Emotional Intelligence: Human Touch: Despite the automation of many tasks, the human touch remains crucial. Customer success professionals will need to balance AI-driven insights with empathy, emotional intelligence, and interpersonal skills to build and maintain strong customer relationships. Conflict Resolution: AI can provide data and predictions, but resolving complex issues and conflicts will still require a skilled customer success professional's nuanced understanding and personal touch. 5. Personalization at Scale: Tailored Strategies: AI enables personalized customer experiences by analyzing individual customer data and preferences. Customer success professionals can leverage these insights to develop tailored strategies for different customer segments. Scalable Personalization: AI allows for personalization at scale, ensuring that even as the customer base grows, each customer receives a personalized experience. 6. New Roles: Some customer success professionals may take on roles focused on managing and optimizing AI tools, ensuring they are configured and used effectively to meet business goals. 7. Continuous Learning and Adaptation: As AI technology evolves, customer success professionals will need to stay updated on the latest advancements and continuously adapt their strategies and workflows. Embracing a mindset of lifelong learning will be essential, as ongoing education and training will be necessary to keep pace with AI advancements and and stay relevant as professionals.
...Read More559 Views
1 request