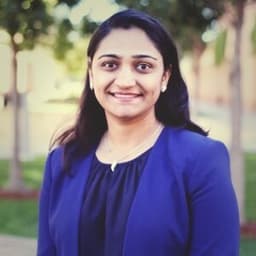
AMA: DocuSign Director of Product Management, Hiral Shah on AI Product Management
May 7 @ 10:00AM PST
Register for AMA
DocuSign Director of Product Management, Hiral Shah on AI Product Management
We will email you Hiral's answers
to these questions after the event in case you can't make it.
Questions must be topic related and not promotional. (250 character limit)
Top Questions
What's your framework to prioritizing needs/deliverables when you're the first Product Manager at a company establishing the function?
What are the different types of AI Product Managers. Are we going to see a role that's similar to a Technical Product Manager focused towards the data science team?
My question is more to understand if a PM needs to understand the AI concepts to be a successful AI PM?
What metrics do ML product teams look at to define success? Which do you find to be the most important?
Who is better suited for an AI product management role compared to a traditional pm role?
How to get a first entry level job in the AI PM field? I have a strong software engineering background but I am interested in the AI PM role. How to make this career change?
What are the challenges in implementing an ML feature in a product? who takes the decision to determine what type of ML feature would help the product and how to solve the problem related to it? How closely AI PM works with Data scientists?
Have you received user feedback regarding the AI features, and how are you incorporating it into product improvements?
What strategies are you using for testing and validating the AI components of your product?
How do you handle scenarios where the AI may not perform as expected?
How do you assess the impact of AI on overall product performance and user engagement?
What emerging trends in AI and machine learning are you closely monitoring for potential integration into your product?
Have you encountered any challenges in terms of user understanding or acceptance of AI?
What is the one thing you advice an AI PM to have? How should they differentiate themselves amongst other PMs?
Work At DocuSign
Senior Product Manager - Payments in San Francisco, California | DocuSign
DocuSign
View job
Upcoming AMA's
Show All