What are some best practices for managing data quality for RevOps reporting?

For internally generated data, I work with my team and the sales leaders to (1) set clear expectations, (2) reinforce the expectations, and (3) make it as easy as possible to meet the expectation. For external data sources, we work closely with other teams (Marketing Ops, Finance) to optimize our subscriptions and evaluate different data sources each year.
Internal data
At Intercom, we defined a set of rules around opportunity management that every rep and manager understands. We're always revisiting this depending on the priorities of the business and changes to the sales process, but we try to be clear with everyone what is required. Of course not everyone follows it perfectly, but this gives us a target to work toward.
To reinforce this expectation, we have done two formal things. First, my team sends our a weekly hygiene report that shows the number of "errors" across approximately 10 fields we care most about and believe drive rigor in the deal process. This gives everyone in the org a view of data quality. Second, we recently started a weekly pipeline hygiene meeting, led by each manager with their team, as a Friday afternoon "let's clean everything up". That has shown marked improvements in hygiene on essentials like close date, expected ARR, stage, next meeting date, etc. that can get lost if this is left to reps on their own with a suggestion to "do your hygiene".
For the third part, we continually review the CRM fields we care most about and deprecate obsolete ones and seek ways to streamline them. For example, we recently changed our competitor tracking fields to have a clearer picture. Throughout that update, we sought ways to make it easier for reps to complete the fields (e.g., pick lists, reminders, auto-populated answers) and put in barriers to move to the next stage to reinforce the most important information. We also allow reps to edit CRM fields directly through Clari and are evaluating Rattle at the moment which has similar functionality. Hygiene will improve when it's easy to do where the reps are already working vs. making it a separate activity to be done.
External data
We also use external data sources to provide insights such as ZoomInfo, D&B, and 6Sense. It's important to understand the strengths and weaknesses of these various external sources, use them appropriately, and set up the right data governance. Sales Ops, Marketing Ops, and Finance at Intercom all collaborate to find the most cost-effective way to get quality 3rd party data into our systems and then make sure it is up-to-date, accurate, and not over-written by users.

One of our first steps was to execute a comprehensive analysis of Data Quality issues, starting with our business use-cases. We then benchmarked our current state against industry standards, such as the DAMA framework. This highlighted opportunities for LinkedIn to improve both over the short-term and the long term, along with improving our data quality rules, such as reducing both false positives and false negatives. Of that effort, 3 items popped stood out:
Latency Improvements - We remedied our 2-3 days of latency and the information wasn’t actionable for our operations - this was remedied via dedicated effort from Revenue Operations’ technical teams along with engineering.
Erroneous Records & Incomplete Data Sets - On the surface, this might seem like an easy fix, but like most things the devil was in the details. Our data quality engine at the time did not detect issues that were masked in aggregate, so we added rules at deeper levels of granularity.
Sustainability → We also hired a Triaging team to maintain and evolve our ecosystem. Data Quality rules are great, but to build a solution that lasts, it’s also important to implement rules at the systems of record to prevent the creation of bad data.

LinkedIn Vice President of Global Sales Operations, SaaS, Akira Mamizuka on RevOps Reporting

Cisco Senior Director, Global Virtual Sales Strategy and Operations, Ignacio Castroverde on RevOps Reporting

Intercom VP, Sales Operations, Tyler Will on RevOps Reporting
Top Revenue Operations Mentors
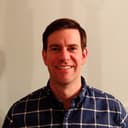
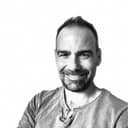
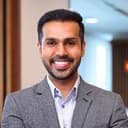
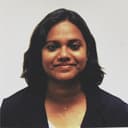

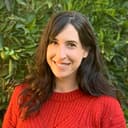
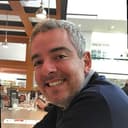
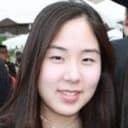
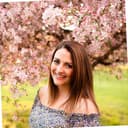
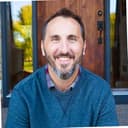