What are the challenges in implementing an ML feature in a product? who takes the decision to determine what type of ML feature would help the product and how to solve the problem related to it? How closely AI PM works with Data scientists?
While infusing ML into a product can be extremely powerful to light-up new scenarios for customers and to optimize current scenarios, it can take a lot of effort to get it right. It all starts with defining and prioritizing the right scenario/problem to focus on, working with data science and ML engineering teams to map the business problem to an ML problem, trying out to different approaches to identify the right one to go ahead with, experimenting and optimizing and then operationalizing the approach and shipping it as part of product stack working with ML engineering and development teams. In addition to focusing on functional aspects and non-functional aspects like performance/scale/reliability, ML scenarios bring in an additional critical aspect of transparency and fairness which is broadly classified under Responsible AI - this is required to gain customer trust and hence feature adoption.
It is the responsibility of product manager to define the business scenarios and success metrics to focus on, work closely with data science teams to map that into ML problem and enable them with right data for them to come up with right approach. Then work with ML engineers and developers to operationalize it as part of the product. In summary, it take collaboration between multiple teams/roles in order to ship great ML features as part of a product.
If you are interested in learning more, watch my session on AI/ML Product Management: https://www.linkedin.com/video/event/urn:li:ugcPost:6929801568753971200/
The first challenge is actually self-imposed by product teams. Ask yourself: are we implementing an ML feature mostly because of FOMO / not wanting to "fall behind", or because of a first-principled, customer-first assessment of need/opportunity?
Assuming we've identified a real need/opportunity for ML in our product, we can ask what type of ML feature best solve the job to be done for our customers.
IMO this is the PM's responsibility to own. It's important to involve and partner with R&D as early as possible to scope the technical challenges of different solutions, but it's unrealistic to expect them to own the step before i.e. identify and define the problem-solution set for the PM.
Another aspect to this is I'd also ask whether the solution ought to be incremental or a fundamental/significant shift from our current set of features and workflows.
You don't want to invest in building incremental ML features (and the engineering/architecture decisions that come with it) only to realize 6-12 months later that you needed a much deeper overhaul, or vice versa.
For example, let's say your app provides a set of dashboards and search functionality to help users find insights in their data. A well-executed conversational interface powered by AI may make it an order of magnitude faster for a user to get the insights or answers they need compared to the status quo, but it'd require a significant overhaul of how users interact with your app today among other potential large decisions e.g. around infra.
Championing such a large overhaul as a PM will almost always require a significant executive buy-in. But if there is a genuine business case to be made, and you throw your hat in the ring to help execute it, it could be a massive career accelerant for you.
Implementing an ML feature vs a non ML feature is no different. The complexity is how the ML model is integrated into the existing product, business or user workflows, and how it might impact the overall end user experience. The goal is usually to simplify and not complicate things. The goal is to reduce buttons and not add buttons. The decision for ML features in a product is similar to the other features -- ML is just the technology about how - under the hood. It is not the end in itself.
As regards working with data scientists -- it depends on the feature. As PMs where there might be significant impact on end user experience, it is helpful that PMs keep in mind the ethics, diversity and inclusion issues to inform data scientists about what to include when capturing data for training and testing. It is important to have a good diverse test data set.
Top Product Management Mentors



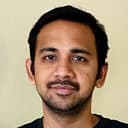


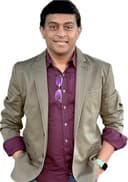

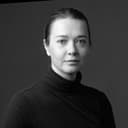
