What methods do you use to forecast demand for new products or features?
Forecasting demand for new products or features requires a blend of quantitative data and qualitative insights. Methods like market research, customer surveys, pilots, historical data, and predictive analytics help outbound PMs reduce uncertainty and make informed forecasts.
-
Market Research and Trend Analysis
Example: Apple’s Launch of AirPods
Apple identified the growing trend of wireless technology and shifting consumer habits toward convenience, using market research to predict strong demand for AirPods.Approach: Analyze industry reports, competitor trends, and emerging technologies to gauge demand for new products.
Action: Use tools like Gartner or IDC reports to benchmark trends and identify market demand signals. Track search trends and social media mentions to validate emerging opportunities.
-
Customer Surveys and Feedback
Example: Dropbox Business Expansion
Before expanding its product from personal use to business, Dropbox conducted customer surveys to understand if existing users would pay for team collaboration tools.Approach: Use surveys, focus groups, and interviews to collect quantitative and qualitative feedback from target customers.
Action: Run email surveys or in-product polls asking customers if they would use or pay for a new feature. Use Net Promoter Score (NPS) data to identify enthusiastic customers who might demand new offerings.
-
Pilot Programs and Beta Testing
Example: Tesla’s Full Self-Driving Beta
Tesla released its autonomous driving software to a select group of drivers to assess demand and collect feedback.Approach: Launch a pilot or beta program to a small group of customers. Measure usage rates, adoption, and feedback to determine market appetite.
Action: Track KPIs like feature engagement and satisfaction scores during the pilot to forecast broader demand upon full release.
-
Sales Forecasting Based on Historical Data
Example: Microsoft Office 365 Subscription Model
When Microsoft transitioned to a subscription model, it used historical data from earlier product sales to predict customer migration and demand for the new model.Approach: Use sales trends, adoption patterns, and growth curves from similar products or features to forecast demand for the new offering.
Action: Analyze existing customer behavior to identify segments likely to adopt the new product and use historical sales data to estimate future demand.
-
Conjoint Analysis and Willingness-to-Pay Studies
Example: Uber’s Dynamic Pricing Model
Uber used conjoint analysis to understand how much users value features like shorter wait times and willingness to pay surge pricing during peak hours.Approach: Use conjoint analysis to determine how customers trade off features, benefits, and price points, helping forecast demand at different pricing tiers.
Action: Conduct conjoint surveys where customers rank preferences for various product configurations, then model demand based on the results.
-
Pre-Orders and Early Sign-Ups
Example: Tesla Cybertruck Pre-Orders
Tesla used pre-order campaigns to gauge market interest in its Cybertruck, collecting deposits to forecast production needs.Approach: Offer pre-orders, waitlists, or early access programs to measure interest before launch.
Action: Monitor the number of pre-orders and early sign-ups as leading indicators of demand.
-
Sales Team Feedback and Channel Insights
Example: Salesforce Lightning Adoption Forecast
Salesforce gathered insights from its sales teams and partners to forecast customer interest in migrating to the new Lightning platform.Approach: Collect input from sales teams, partners, and channel data to forecast demand. Frontline salespeople often have valuable insights into customer interest.
Action: Hold regular feedback sessions with sales and partner teams to capture signals of market readiness and customer demand.
-
Predictive Analytics and Machine Learning Models
Example: Amazon’s Inventory Forecasting for New Products
Amazon uses machine learning models that consider historical sales, seasonality, and external factors to forecast demand for new products.Approach: Use predictive analytics tools to identify correlations and patterns in customer behavior, pricing, and market trends.
Action: Build predictive models based on internal data (past sales, web traffic) and external signals (market trends, economic factors) to estimate future demand.
Top Product Management Mentors
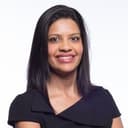


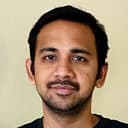



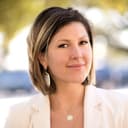

